On Nov 6th, 2023, almost a year after its ChatGPT was released to public, OpenAI hosted its first ever developer conference in San Francisco. Honestly, I haven’t been this pumped about a tech release since the days of Steve Jobs – and let me tell you, there’s a solid reason for that excitement.
At the event, OpenAI presented a whole bunch of cool updates and new features for ChatGPT and other parts of their platform. You can dive into the details of these updates here. But for this article, I’m zeroing in on one particular game-changer: Custom GPTs. I’ll break down (1) why they’re a big deal, (2) how they exemplify an even bigger shift in the AI landscape, and (3) what implications they have for us.
I. What is Custom GPTs and why is it a big deal?
What is Custom GPTs
Unlike ChatGPT, a versatile, general-purpose tool suitable for a wide range of tasks like seeking advice, looking up definitions, editing essays, or coding assistance, Custom GPTs (or simply GPTs) are tailored versions of ChatGPT, each designed for a specific purpose. They provide relevant, precise, and domain-specific responses more efficiently, compared to a general-purpose GPT. Think of it like this: ChatGPT is like an encyclopedia, offering a broad overview on many topics, while Custom GPTs are like specialized books, each focusing in-depth on a specific subject.
What's so novel about Custom GPTs, you might wonder?
They're incredibly user-friendly: even those with no coding background can effortlessly create a Custom GPT for their specific needs.
The creation process is fast and efficient, often taking just minutes or hours.
If you're not keen on making your own, no worries – you can simply visit OpenAI’s equivalent of an App Store and choose one that fits your requirements.
II. How GPTs exemplify an even bigger shift in the AI landscape
Let's take a real-world example to better understand how GPT models are changing the AI game. Picture this task: we want to categorize a bunch of different dishes into their correct food groups. And let's say we've got everything we need for this task: complete dish-related data and their corresponding category labels.
Without GPTs
Traditionally, in situations like this, we'd lean on Machine Learning algorithms, particularly Supervised Learning. But this route is pretty demanding and time-consuming in several ways:
Initial Requirements
(a) lots of data,
(b) all of it labeled, and
(c) top-notch (i.e., expensive) professionals to build the classifier.
Data Prep
The Training & Testing Drill (well ... there are even more steps here, expand if you want to see)
Crafting a complete classifier in this scenario can take anywhere from a few days to weeks for a proper 'toy' model, and it might stretch out to several months for a full-fledged, production-grade model.
With GPTs
Now, let's tackle the same task using a Custom GPT. We're going to up the ante this time by using just the dish names as input for our classifier. That means no extra info like restaurant names, locations, or dish prices. Our goal? To sort these dishes into six categories: đồ uống, món nước, món khô, ẩm thực trung hoa, ẩm thực việt nam, and salad.
Video #1 - Create a Cuisine Classifier GPT
In Video #1, you can see how I easily customized the GPT for my needs using plain, everyday language – a cool thing called prompt engineering. I didn't have to sweat over detailing every aspect of the app I wanted upfront. The GPT was savvy enough to ask for any clarifications or extra instructions it needed. It then boiled down our entire chat into a neat paragraph of specs. And the end result? A model that works surprisingly well, especially given that it only took me under 10 minutes to set up.
Video #2 - Review the Configuration and Test the Cuisine Classifier GPT
So ... what's the big shift?
Before GPTs entered the scene, the norm was to train specific models on task-specific dataset to solve defined tasks. Companies of all sizes often find themselves having a multitude of tasks where AI assistance would be beneficial–something that's incredibly costly to realize. And even with an unlimited budget, two major concerns remain: firstly, whether the company has enough data available to train a model that's truly effective, and secondly, how to attract skilled data scientists, especially for organizations outside the tech or finance sectors.
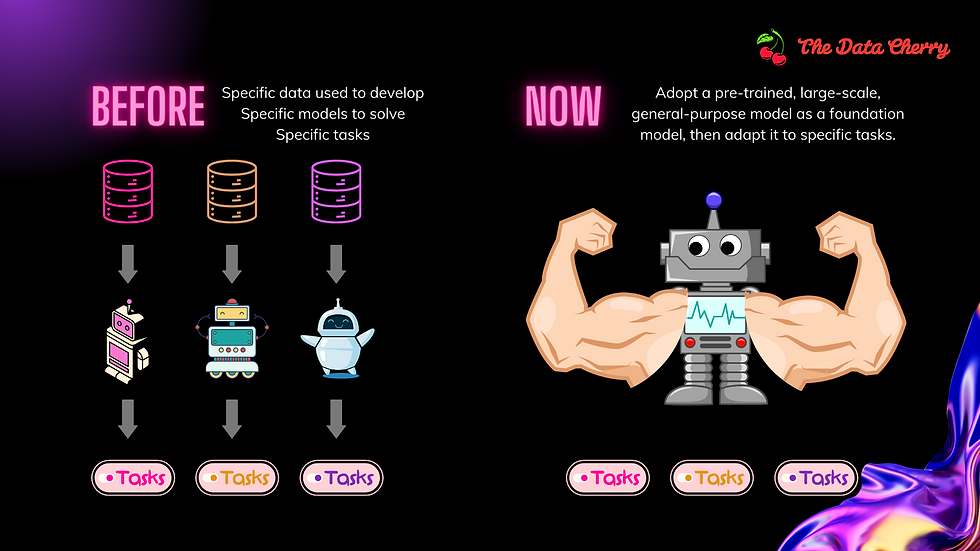
When OpenAI introduced Custom GPTs, essentially they allow people to adopt their state-of-the-arts, large-scale, general-purpose GPT as a foundation model, based on which people can further adapt it to their specific tasks at very little additional cost in term of time, money and effort. As our example illustrates, using Custom GPTs to build a model is far less laborious than the traditional approach, particularly in three critical areas: initial requirements, data preparation, and the training and testing phases. This difference is a game-changer, especially for companies in less consumer-focused industries that previously found it challenging to tap into the benefits of data science and analytics due to these hurdles.
If you are interested in the more technical aspect, I would suggest looking into out Retrieval-Augmented Generation (RAG). It is a method that combines the strengths of pre-trained language models with a retrieval system. Essentially, it retrieves more relevant and up-to-date documents or data then uses this information to enrich and inform (augment) the generation process of the language model.
III. What does that mean to us?
For businesses
AI applications of high quality are no longer exclusive to wealthy, data-rich tech and finance companies. They are now readily available for customization to suit various use cases at a significantly lower cost and time investment. This democratization of technology is poised to boost productivity and improve business performance across the board.
For entrepreneurs/developers
Yes, some companies were basically dead or faced serious competition after OpenAI released their various new features and improvements (e.g., Jasper AI, Grammarly, etc.). However, much like Apple doesn't create every app in its App Store, there remains ample opportunity for entrepreneurs and developers to profit within the GPTs Store. The key is in being strategic about what to build.
In my view, a particularly promising opportunity lies in assisting businesses to tailor these foundational models to their specific use cases. This involves leveraging a company's internal data and integrating applications seamlessly into their existing systems, all while offering these services at a competitive price.
For data professionals
For all you data pros out there, let's get one thing straight: your data know-how is always going to be a huge asset in today's digital world. But let's face the reality: the demand for data-related jobs might slow down. This is due to (1) increased productivity (think GitHub Copilot for developers or Data Analysis GPT), (2) the shift to an adopt-and-adapt approach in AI (e.g., Custom GPTs), and (3) as businesses harness AI to optimize operations (meaning reducing costs while maintaining efficiency — it's about how much you save, not just the job title or count).
Given this context, here's my two cents: the three factors mentioned are poised to rapidly evolve the roles of most data professionals. We're moving from a focus on creation and building to roles that are more akin to architects, editors, or quality controllers. As a result, it's highly likely we'll see the creation of new job roles and titles that align with these changing demands and skill sets.
References
What are Generative AI models? (2023) YouTube. YouTube. Available at: https://www.youtube.com/watch?v=hfIUstzHs9A&t=33s (Accessed: 14 November 2023).
Introducing GPTs. Available at: https://openai.com/blog/introducing-gpts (Accessed: 14 November 2023).
New models and developer products announced at DevDay. Available at: https://openai.com/blog/new-models-and-developer-products-announced-at-devday (Accessed: 14 November 2023).
Comments