Ever wondered, "Why would OpenAI release SearchGPT when it already has ChatGPT?" Or, "ChatGPT can answer almost anything, but would you trust it with the exact steps for returning an order on TikTok?" If these questions make you curious, then dive into my latest 'gentle guide' on #RAG! RAG is an exciting technique that supercharges AI, allowing it to give you more accurate, relevant answers by tapping into up-to-date, external knowledge. 🎯
We’ll start with an Introduction that sets the stage by exploring some real-world questions and challenges—questions that even advanced AI models sometimes struggle with. You’ll see why general language models (LLMs) aren’t always equipped to handle specific, up-to-date queries and how Retrieval-Augmented Generation (RAG) steps in as a solution.
Next, we dive into RAG Systems, breaking down the core components that make this technique so effective. You’ll learn about embeddings, the external knowledge base, and how the processes of retrieval, augmentation, and generation all come together to deliver more accurate responses. We’ll also summarize these components and touch on the key challenges that come with implementing RAG.
Finally, we’ll look at Use Cases—from customer service to search integration and knowledge management. These real-world applications show how RAG can provide reliable, context-aware answers, helping industries make the most of AI.
For the full scoop, explore the interactive slides below (be sure to click the interactive buttons on the upper right!) or, if you prefer a traditional, static view, click here.
INTERACTIVE SLIDES
STATIC SLIDES

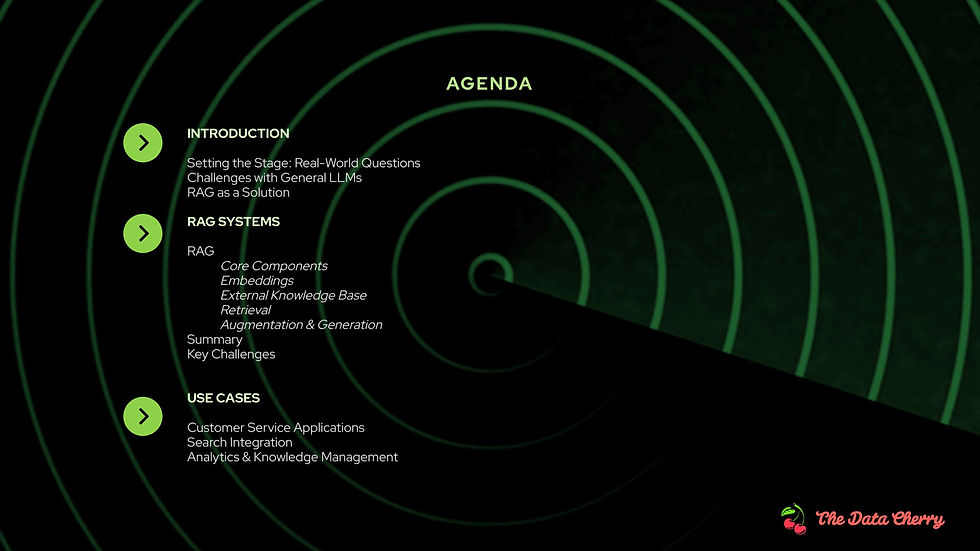
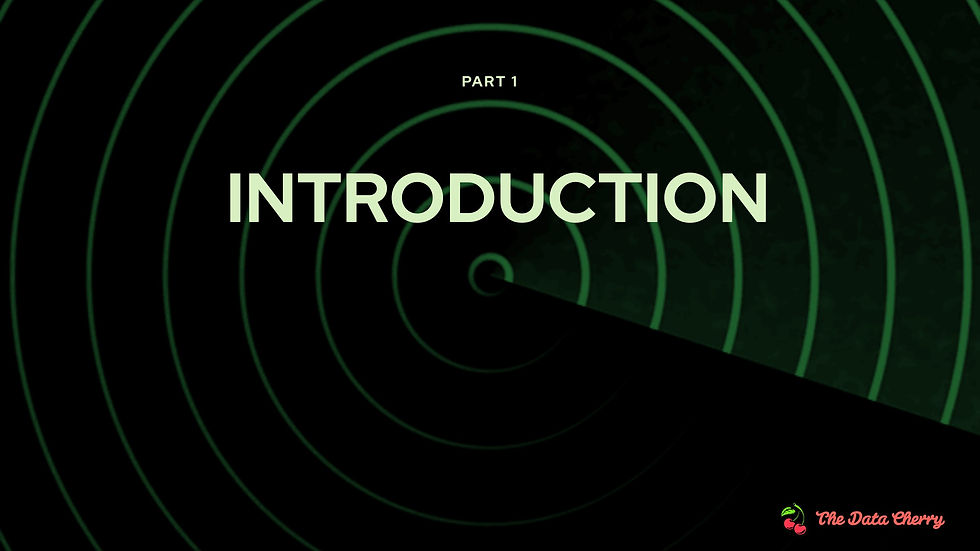
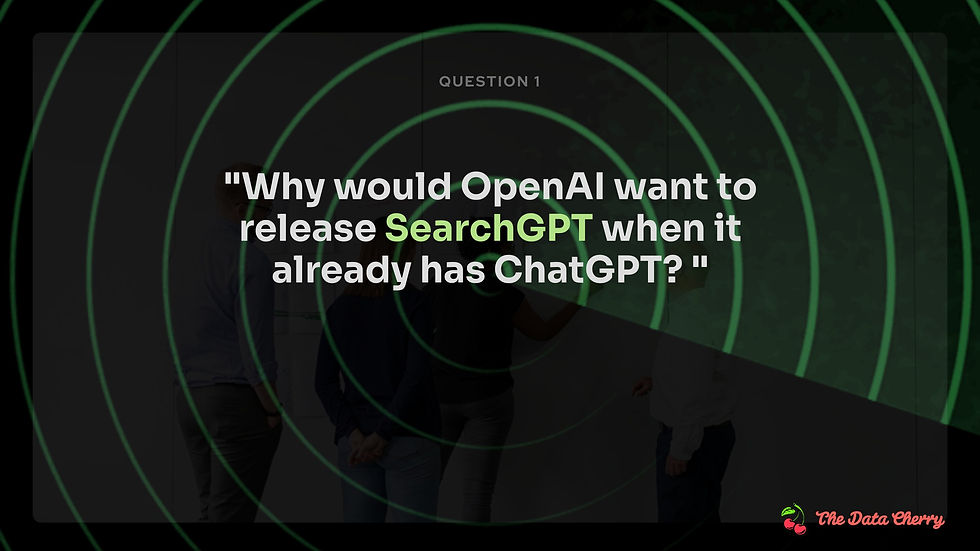
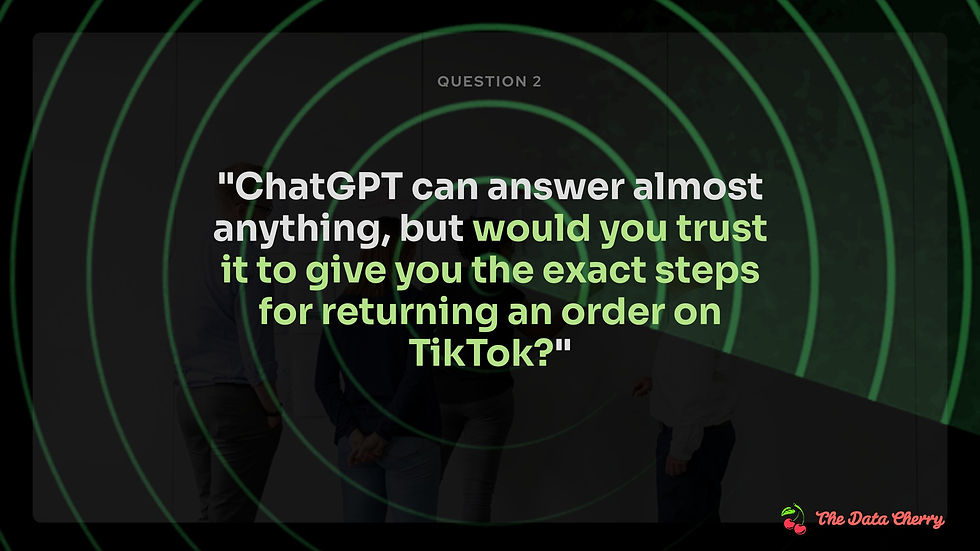
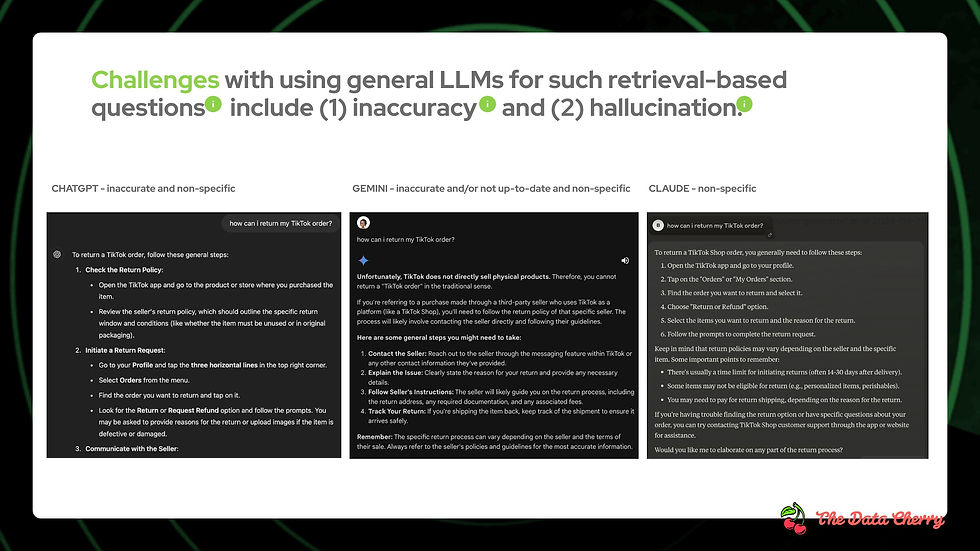
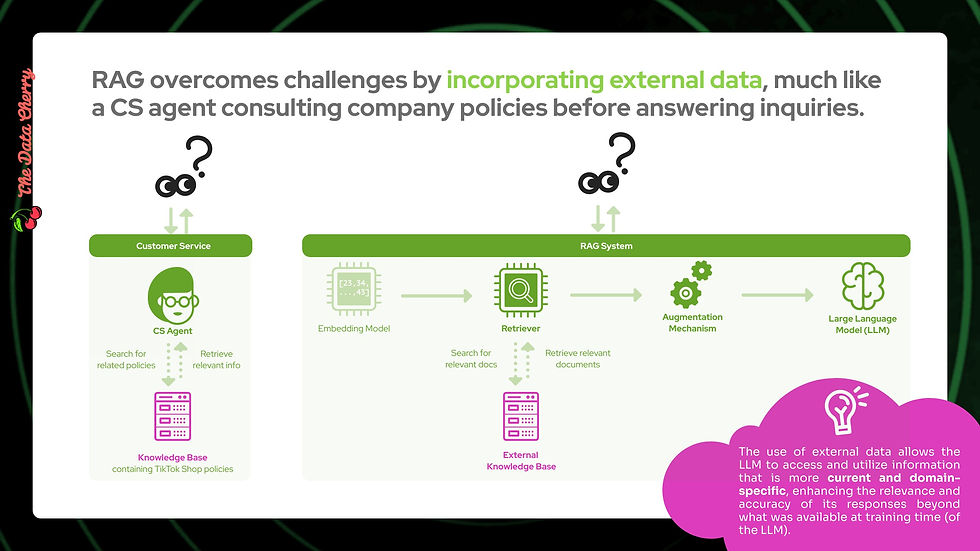
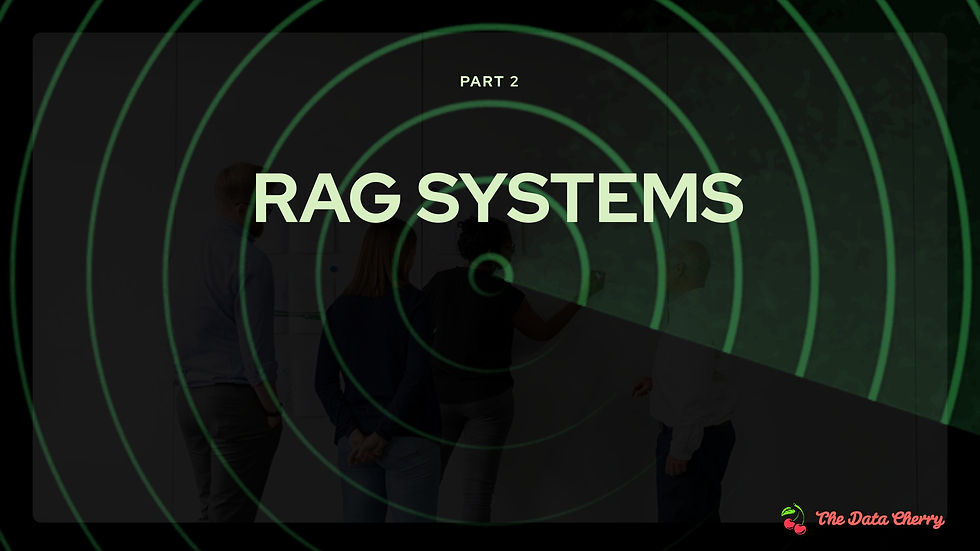
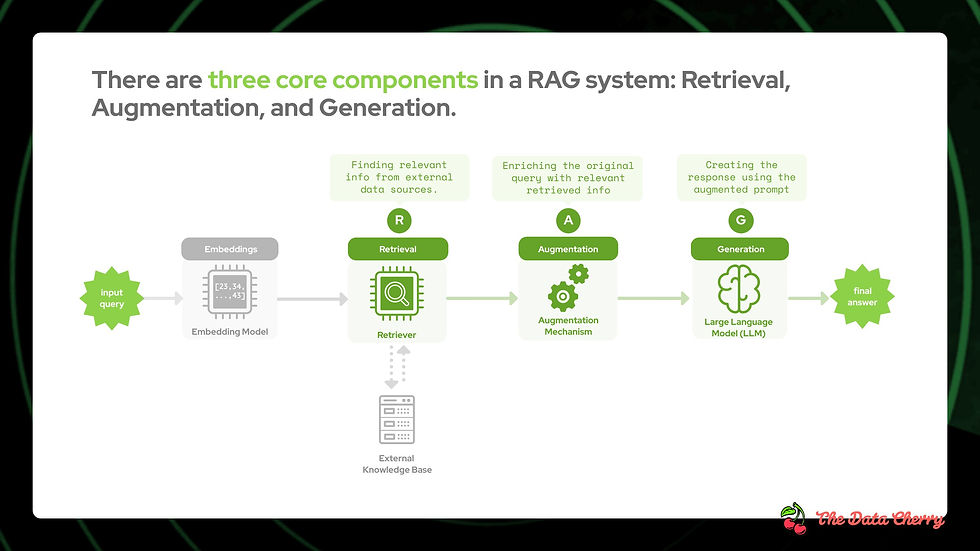
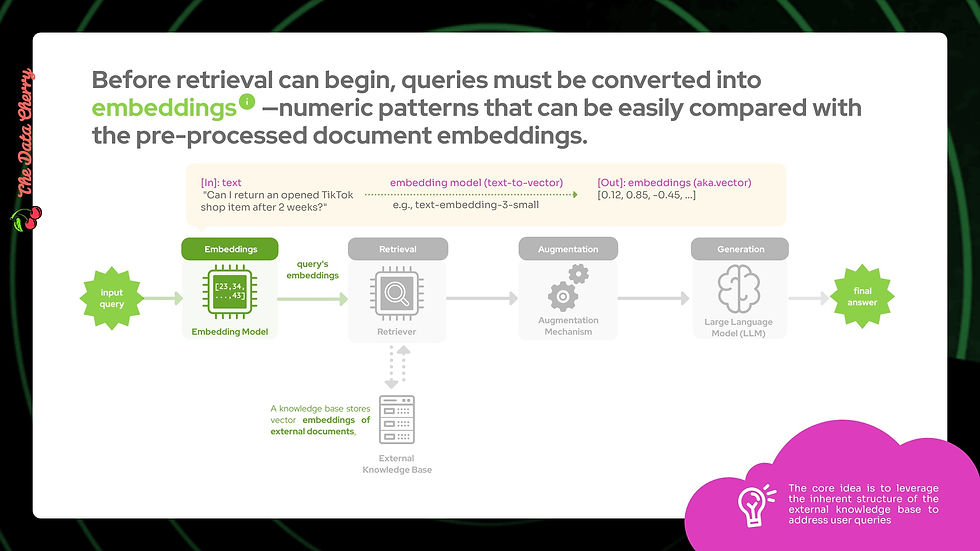

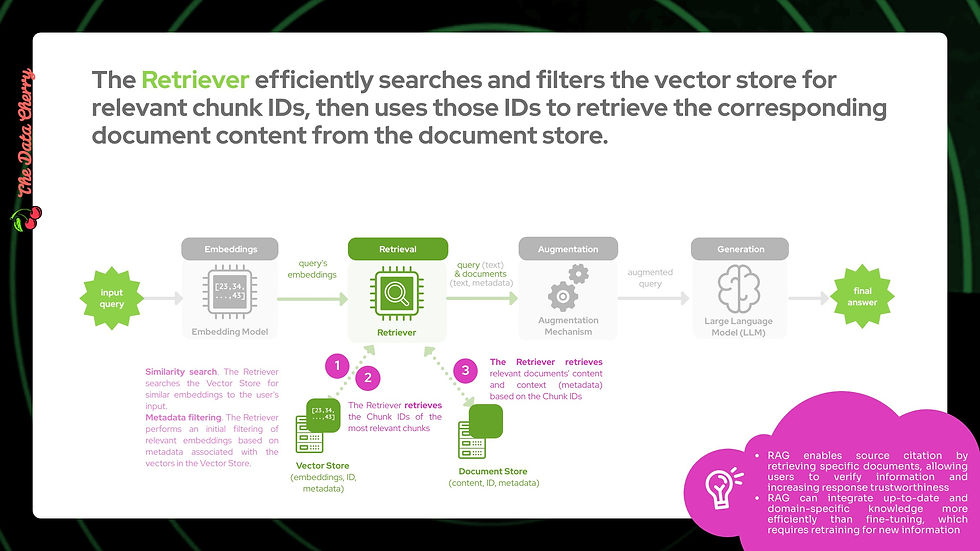
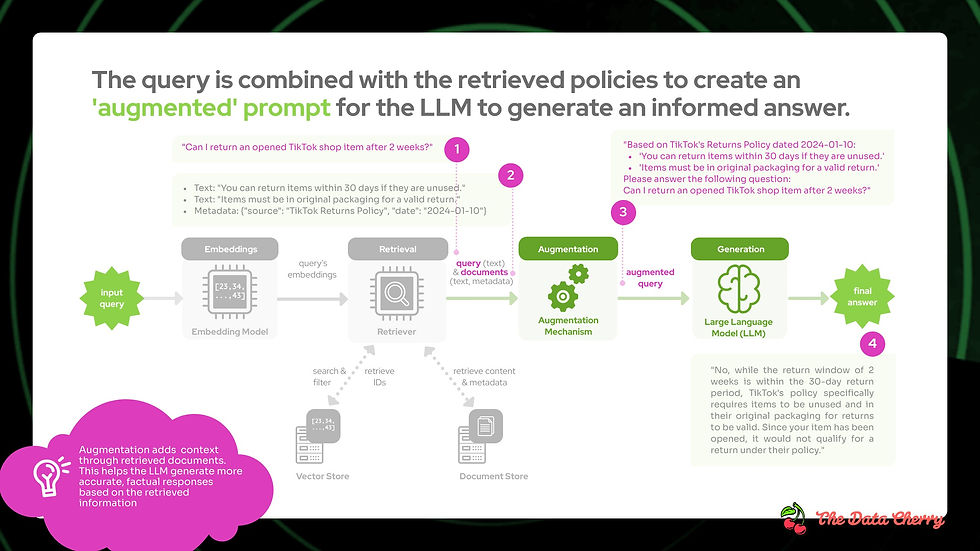
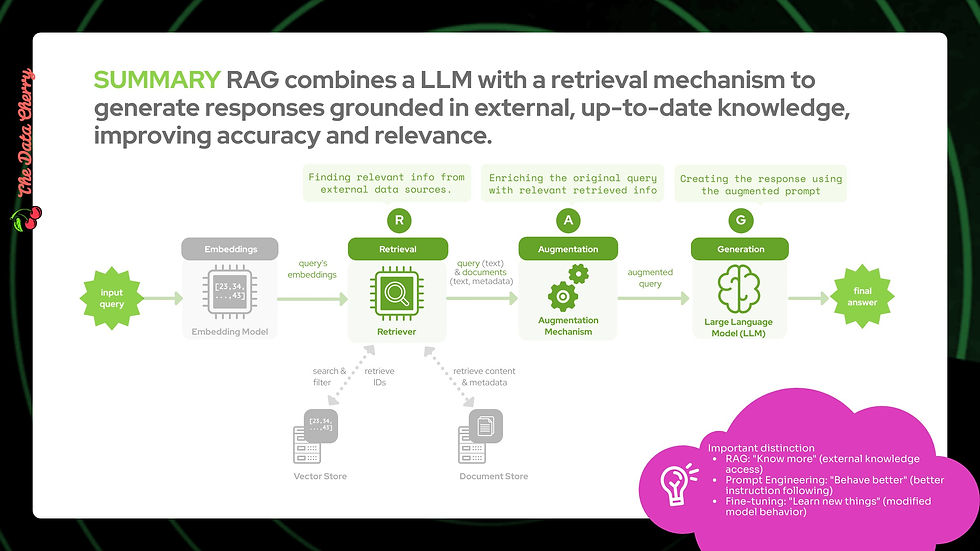

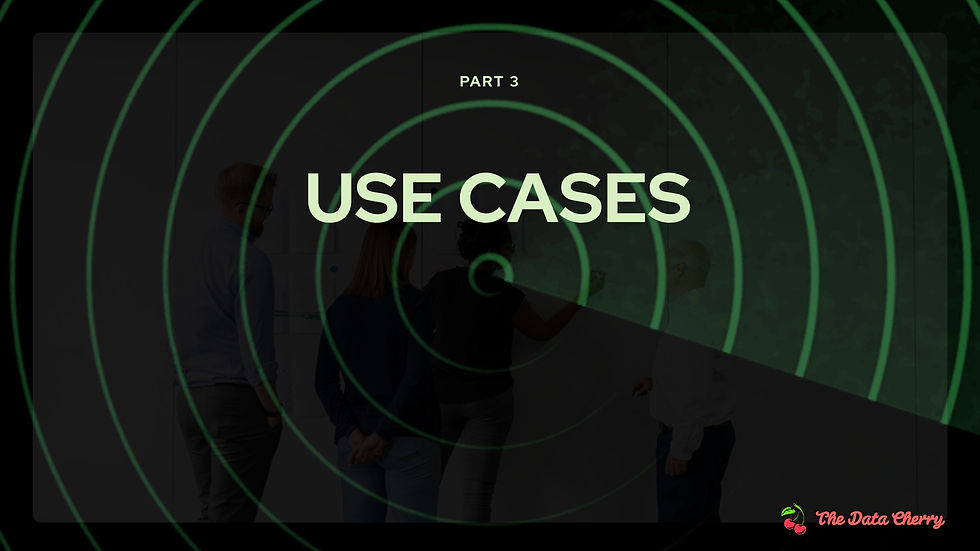


Comments